FEDML Launch - Run Any GenAI Jobs on Globally Distributed GPU Cloud: Pre-training, Fine-tuning, Federated Learning, and Beyond
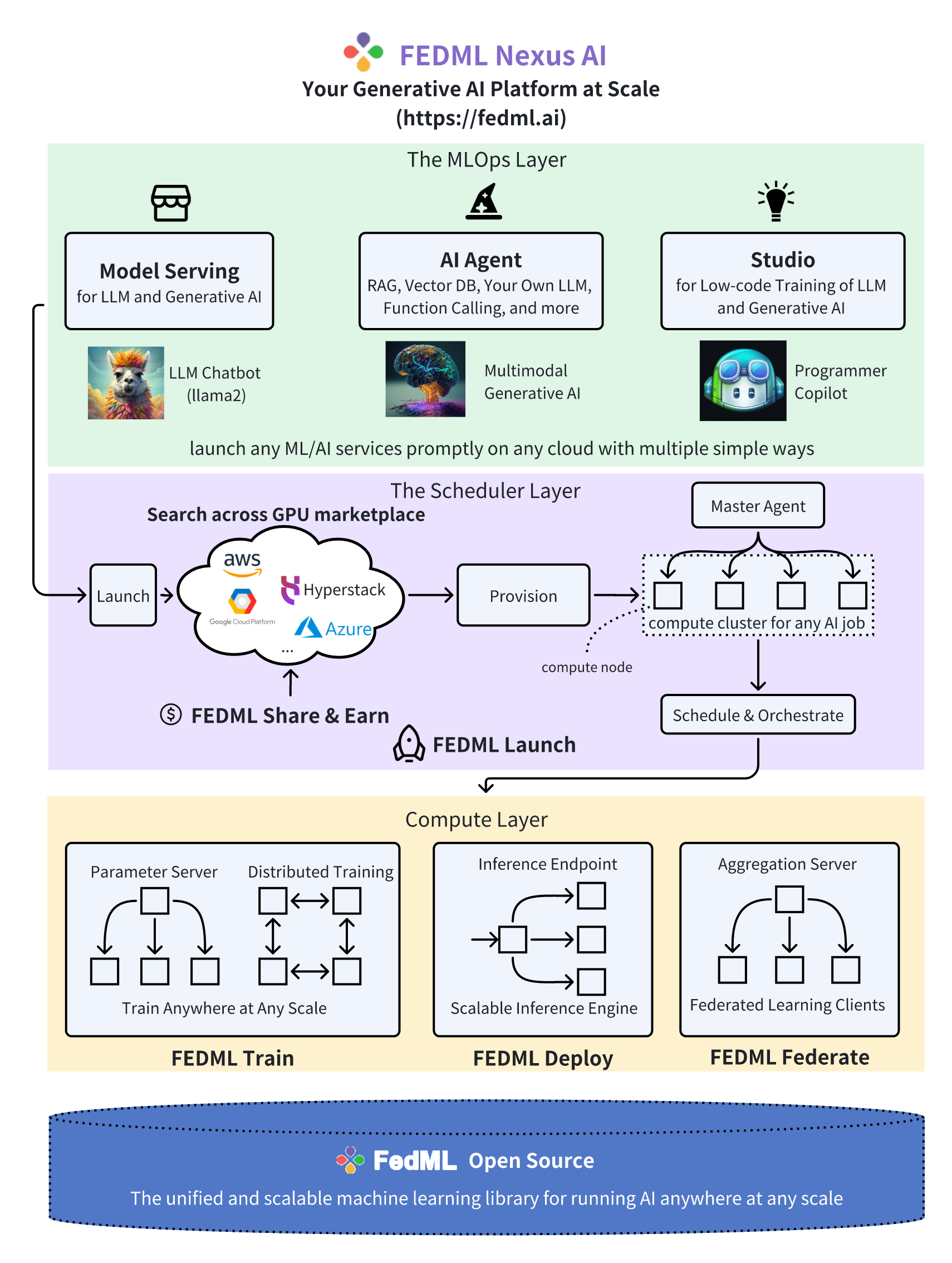
- Mission & Vision
- The Advantages of Distributed AI Platform
- FEDML Launch Overview
- Quick start
- Training as a Cloud Service
- Train on Your own GPU cluster
- Experiment Tracking for FEDML Launch
- Advanced Features: Batch Job and Workflow
- About FEDML, Inc.
Platform: https://fedml.ai
GitHub: https://github.com/FedML-AI/FedML
Documentation: https://doc.fedml.ai
Mission & Vision
Artificial General Intelligence (AGI) promises a transformative leap in technology, fundamentally requiring the scalability of both models and data to unleash its full potential. Organizations such as OpenAI and Meta have been at the forefront, advancing the field by adhering to the "scaling laws" of AI. These laws posit that larger machine learning models, equipped with more parameters and trained with more data, yield superior performance. Nonetheless, the current approach, centered around massive GPU clusters within a single data center, poses a significant challenge for many AI practitioners.
Our vision is to provide a scalable AI platform to democratize access to distributed AI systems, fostering the next wave of advancements in foundational models. By leveraging a greater number of GPUs and tapping into geo-distributed data, we aim to amplify these models' collective intelligence. To make this a reality, the ability to seamlessly run AI jobs from a local laptop to a distributed GPU cloud or onto on-premise clusters is essential—particularly when utilizing GPUs spread across multiple regions, clouds, or providers. It is a crucial step for AI practitioners to have such a product at their fingertips, toward a more inclusive and expansive future for AGI development.
At FEDML, we developed FEDML Launch, a super launcher that can run any generative AI jobs (pre-training, fine-tuning, federated learning, etc.) on a globally distributed GPU cloud. It swiftly pairs AI jobs with the most economical GPU resources, auto-provisions, and effortlessly runs the job, eliminating complex environment setup and management. It supports a range of compute-intensive jobs for generative AI and LLMs, such as large-scale training, fine-tuning, serverless deployments, and vector DB searches. FEDML Launch also facilitates on-premise cluster management and deployment on private or hybrid clouds.
The Advantages of Distributed AI Platform
- Increased Reliability and Availability. By spreading resources across multiple clouds, developers can ensure that if one provider experiences downtime, the model service can still run on the other providers. Automated failover processes ensure that traffic is redirected to operational instances in the event of a failure.
- Scalability. Cloud services typically offer the ability to scale resources up or down. Multiple providers can offer even greater flexibility and capacity. Distributing the load across different clouds can help manage traffic spikes and maintain performance.
- Performance. Proximity to users can reduce latency. By running endpoints on different clouds, developers can optimize for geographic distribution. Different clouds might offer specialized GPU types that are better suited for particular types of workloads.
- Cost Efficiency. Prices for cloud services can vary. FEDML Nexus AI uses multiple providers allowing developers to take advantage of the best pricing models. Developers can bid for unused capacity at a lower price, which might be available from different providers at different times.
- Risk Management and Data Sovereignty. Distributing across different regions can mitigate risks associated with policy regulations affecting service availability. For compliance reasons, developers might need to store and process data in specific jurisdictions. Multiple clouds can help meet these requirements.
FEDML Launch Overview
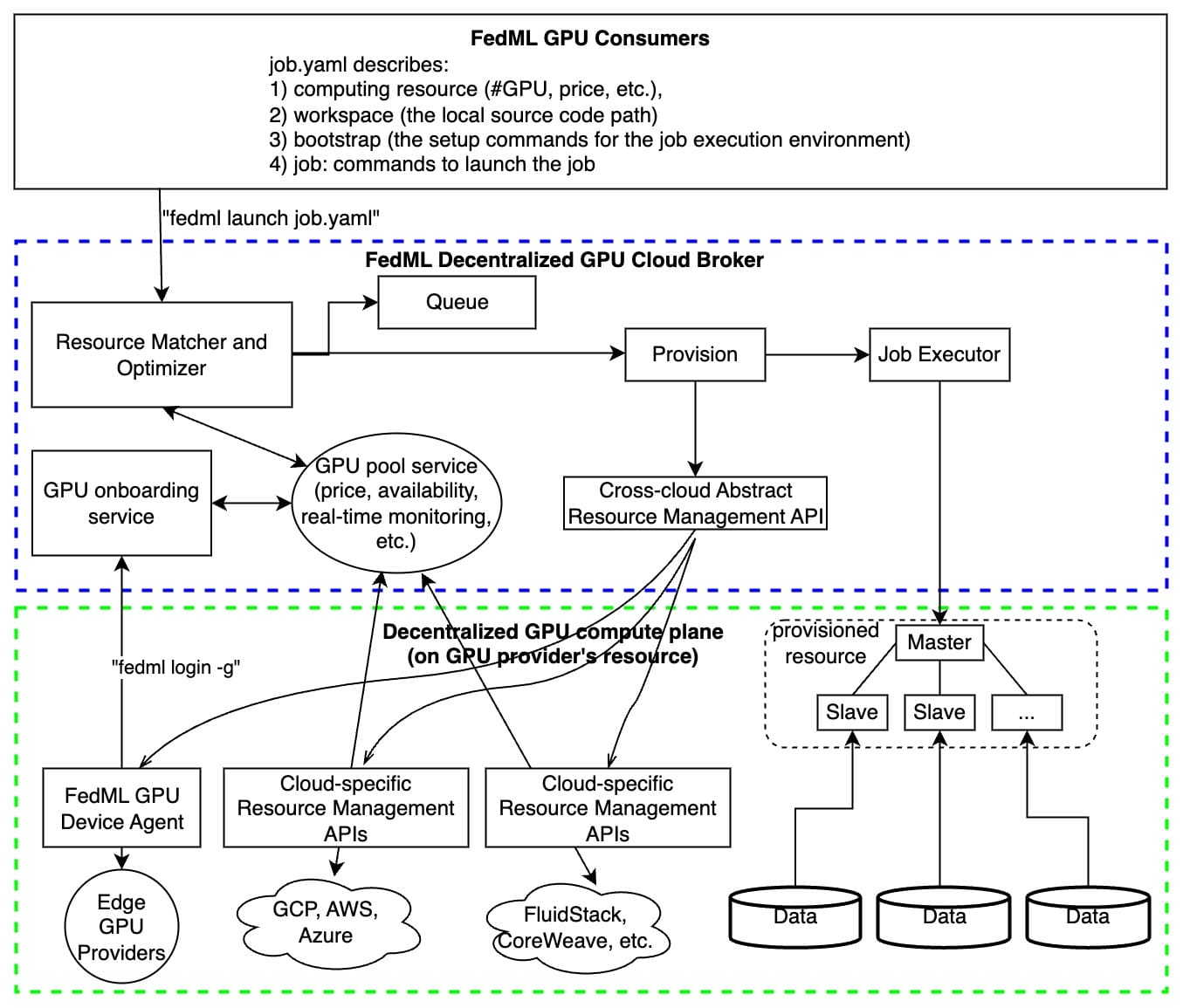
As shown in the figure above, FEDML Launch works as following consecutive steps:
- define ML job without code change in a declarative format (e.g., YAML) or reuse our pre-built job templates
- launch the ML job with just one-line CLI or one-click in GUI
- search for cheaper GPUs across a large number of GPU providers without price lock-in
- provision automatically for GPU resources and the software environment setup tailored for the job
- manage cluster for concurrent jobs with job queue support
- orchestrate your ML job across multi-nodes/geo-distributed environments, it can be model deployment across GPU nodes, distributed training, or even federated learning across clouds.
- run and monitor your job with rich observability features so you can see the real-time billing, metrics, logs, system performances, as well as diagnose performance bottlenecks by fine-grained profiling.
The value proposition of FEDML Launch:
- Find the lower prices without cloud vendor lock-in, in any clouds
- The highest GPU availability, provision in all zones/regions/clouds, even individual GPU contributors from the community
- Define your scheduling strategies to save money or request resources in a higher priority
- User-friendly MLOps to save time on environment management (AI docker hub for developers)
- On-premises GPU cluster management
- Provide Machine Learning as a Service (MLaaS) with Launch: if you have GPU resources, valuable datasets, or even a foundation model and hope to provide cloud service for your own customers to use them as Inference API or Training As a Service, FEDML Launch would be the off-the-shelf enterprise solution for it.
- FEDML Launch is versatile in any AI jobs, including training, deployment, and federated learning. It can also be used for complex multi-step jobs such as serving AI agents, building a customized machine learning pipeline for model continual refinement.
Quick start
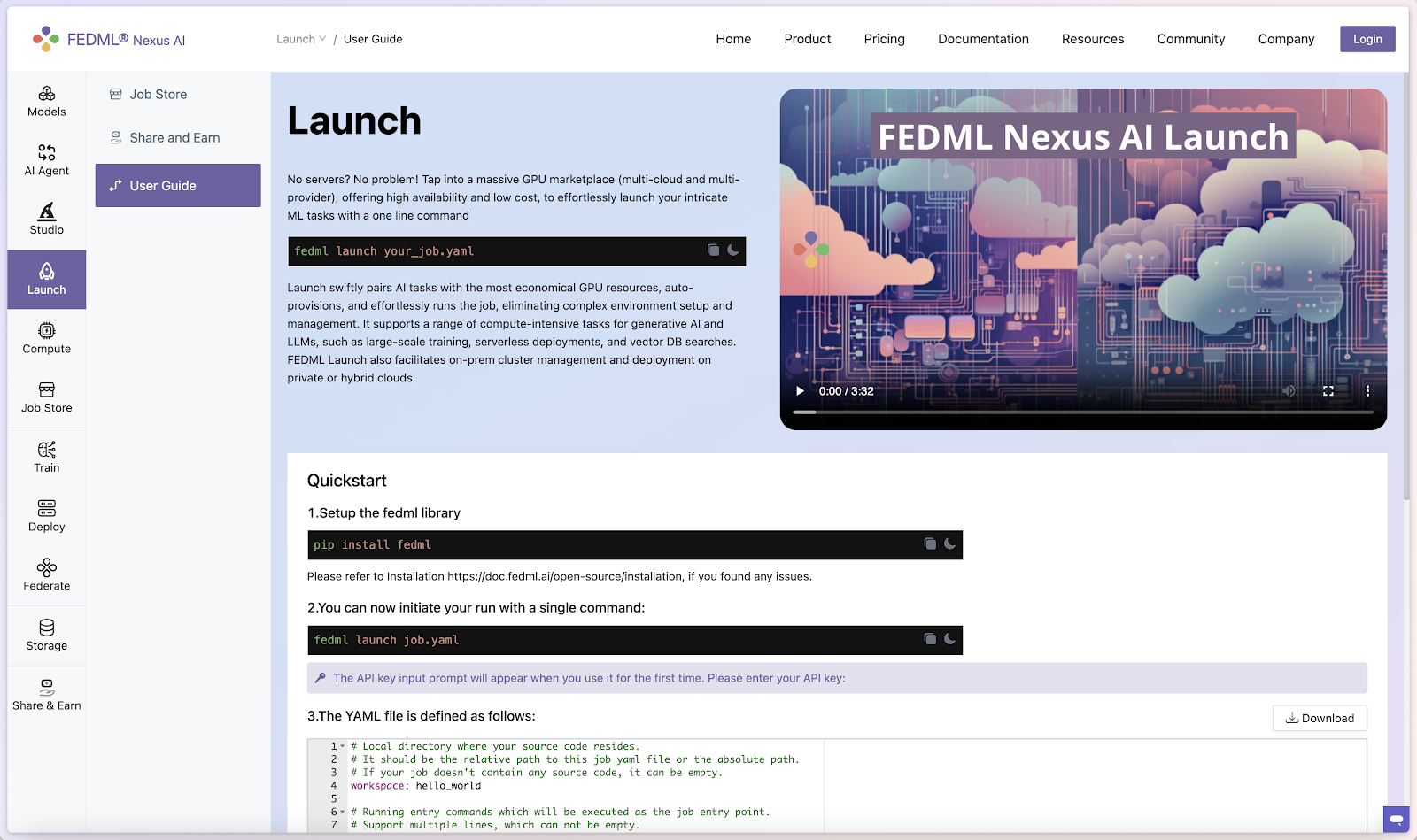
- Set up the FEDML library
Install Python library for interacting with FEDML Launch APIs.
pip install fedml
- Create job.yaml file
Before launch any job, at first, you need to define your job properties in the job yaml file, e.g. workspace, job, bootstrap, etc.
Below is an example of job yaml file:
fedml_env:
project_name: my-project
# Local directory where your source code resides.
# It should be the relative path to this job yaml file.
# If your job doesn't contain any source code, it can be empty.
workspace: hello_world
# Bootstrap shell commands which will be executed before running entry commands.
# Support multiple lines, which can be empty.
bootstrap: |
pip install -r requirements.txt
echo "Bootstrap finished."
# Running entry commands which will be executed as the job entry point.
# If an error occurs, you should exit with a non-zero code, e.g. exit 1.
# Otherwise, you should exit with a zero code, e.g. exit 0.
# Support multiple lines, which can not be empty.
job: |
echo "Hello, Here is the launch platform."
echo "Current directory is as follows."
pwd
python hello_world.py
computing:
minimum_num_gpus: 1 # minimum # of GPUs to provision
# max cost per hour of all machines for your job.
# E.g., if your job are assigned 2 x A100 nodes (8 GPUs), each GPU cost $1/GPU/Hour, "maximum_cost_per_hour" = 16 * $1 = $16
maximum_cost_per_hour: $1.75
resource_type: A100-80G # e.g., A100-80G, please check the resource type list by "fedml show-resource-type" or visiting URL: https://fedml.ai/accelerator_resource_type
For more details and properties about the job yaml file, please refer to job yaml file.
- Launch a job
Launch a job to the GPU Cloud.
fedml launch /path/to/job.yaml
NOTE: Note that you might be prompted for API_KEY the first time you run the command. Please get this key from your account on FEDML Nexus AI Platform. You can also specify the API_KEY with the -k option.
After the launch CLI is executed, you will get the following output prompting for confirmation of resources:
❯ fedml launch job.yaml -v
Submitting your job to FedML® Nexus AI Platform: 100%|█████████████████████████████| 2.92k/2.92k [00:00<00:00, 16.7kB/s]
Searched and matched the following GPU resource for your job:
+-----------+-------------------+---------+------------+-------------------------+---------+------+----------+
| Provider | Instance | vCPU(s) | Memory(GB) | GPU(s) | Region | Cost | Selected |
+-----------+-------------------+---------+------------+-------------------------+---------+------+----------+
| FedML Inc | FEDML_A100_NODE_2 | 256 | 2003.85 | NVIDIA A100-SXM4-80GB:8 | DEFAULT | 1.09 | √ |
+-----------+-------------------+---------+------------+-------------------------+---------+------+----------+
You can also view the matched GPU resource with Web UI at:
https://fedml.ai/launch/confirm-start-job?projectId=1717259066058870784&projectName=my-project&jobId=1717260771043446784
Do you want to launch the job with the above matched GPU resource? [y/N]:
You can either confirm through terminal or may even open the run url to confirm. Once resources are confirmed, it will then run your job, and you will get the following output:
Do you want to launch the job with the above matched GPU resource? [y/N]: y
Launching the job with the above matched GPU resource.
Failed to list run with response.status_code = 200, response.content: b'{"message":"Succeeded to process request","code":"SUCCESS","data":null}'
You can track your run details at this URL:
https://fedml.ai/train/project/run?projectId=1717259066058870784&runId=1717260771043446784
For querying the realtime status of your run, please run the following command.
fedml run logs -rid 1717260771043446784
- Realtime status of your run
You can query the real time status of your run with the following command.
fedml run logs -rid <run_id>
More run management CLIs can be found here
- You can also view the details of the run on the FEDML Nexus AI platform:
Log into to the FEDML Nexus AI Platform (https://fedml.ai) and go to Train > Projects (my_project) Select the run you just launched and click on it to view the details of the run.
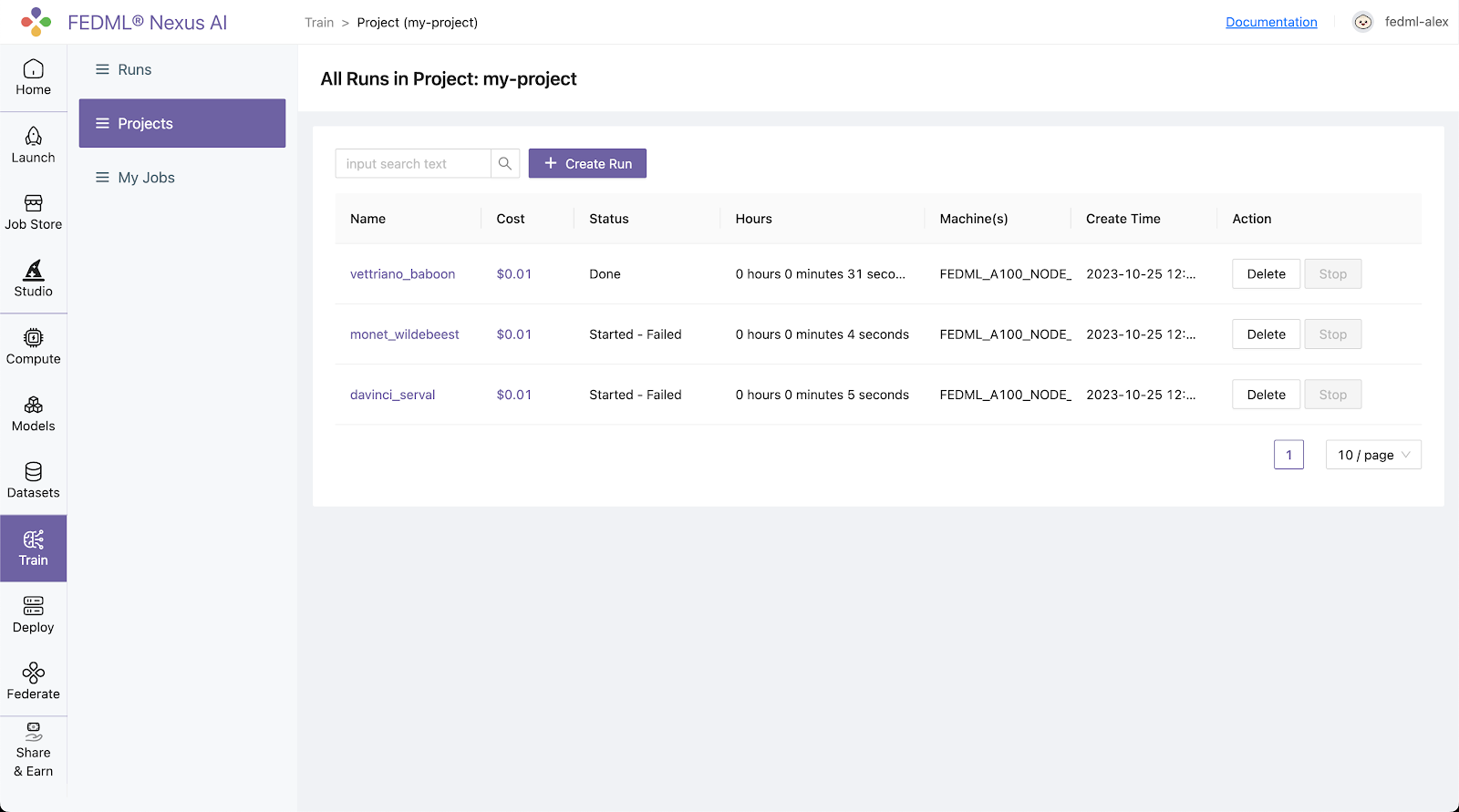
Alternatively, you can also go to Train / Runs to find all of your runs scattered across all of your projects unified at a single place.
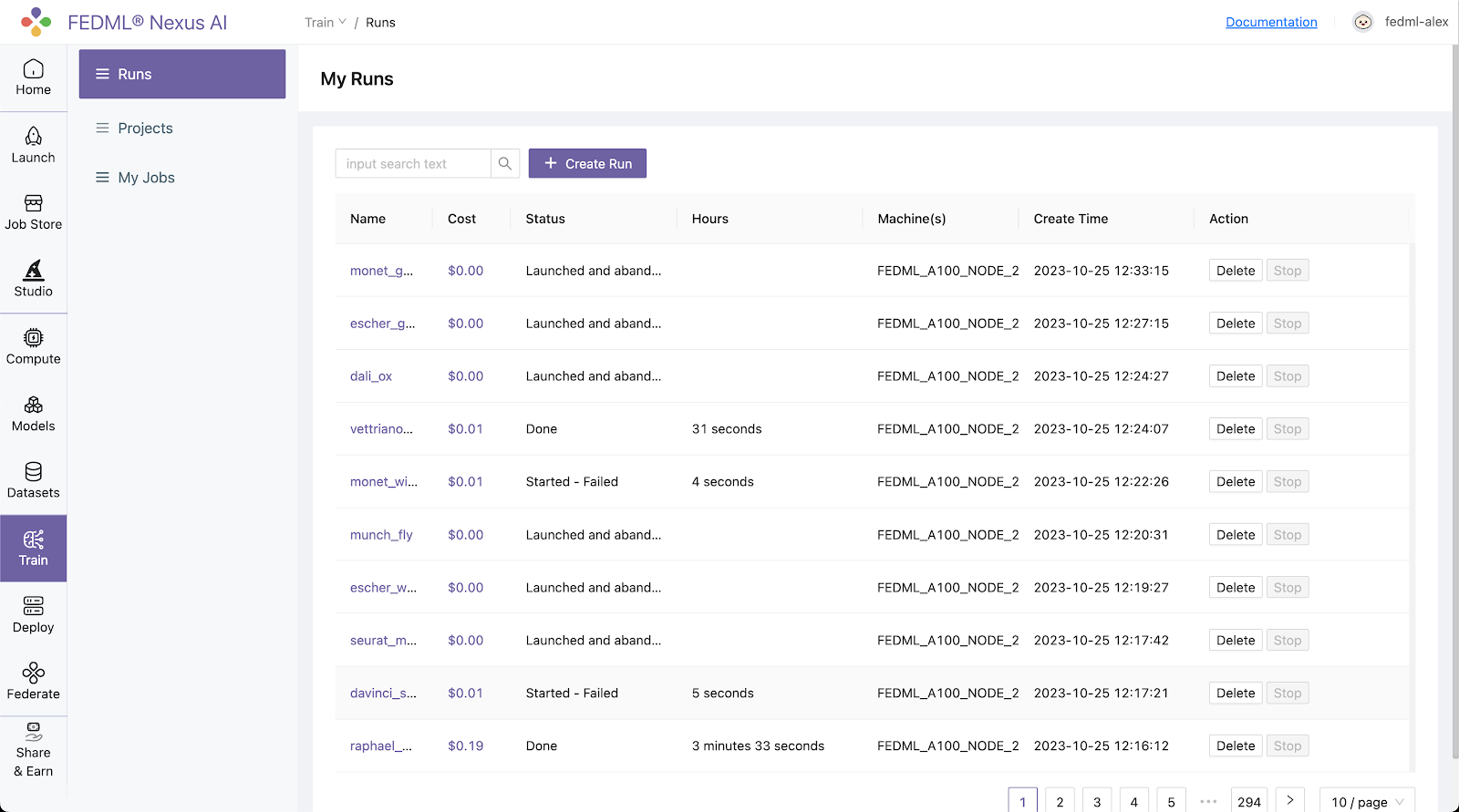
The URL link to FEDML Nexus AI Platform for your run is printed in the output of the launch command for quick reference.
You can track your run details at this URL:
https://fedml.ai/train/project/run?projectId=1717259066058870784&runId=1717260771043446784
For querying the realtime status of your run, please run the following command.
fedml run logs -rid 1717260771043446784
This is the quickest, one-click way to go to your run UI. The Run UI offers a lot of information about your run including Metrics, Logs, Hardware Monitoring, Model, Artifacts, as shown in the image below:
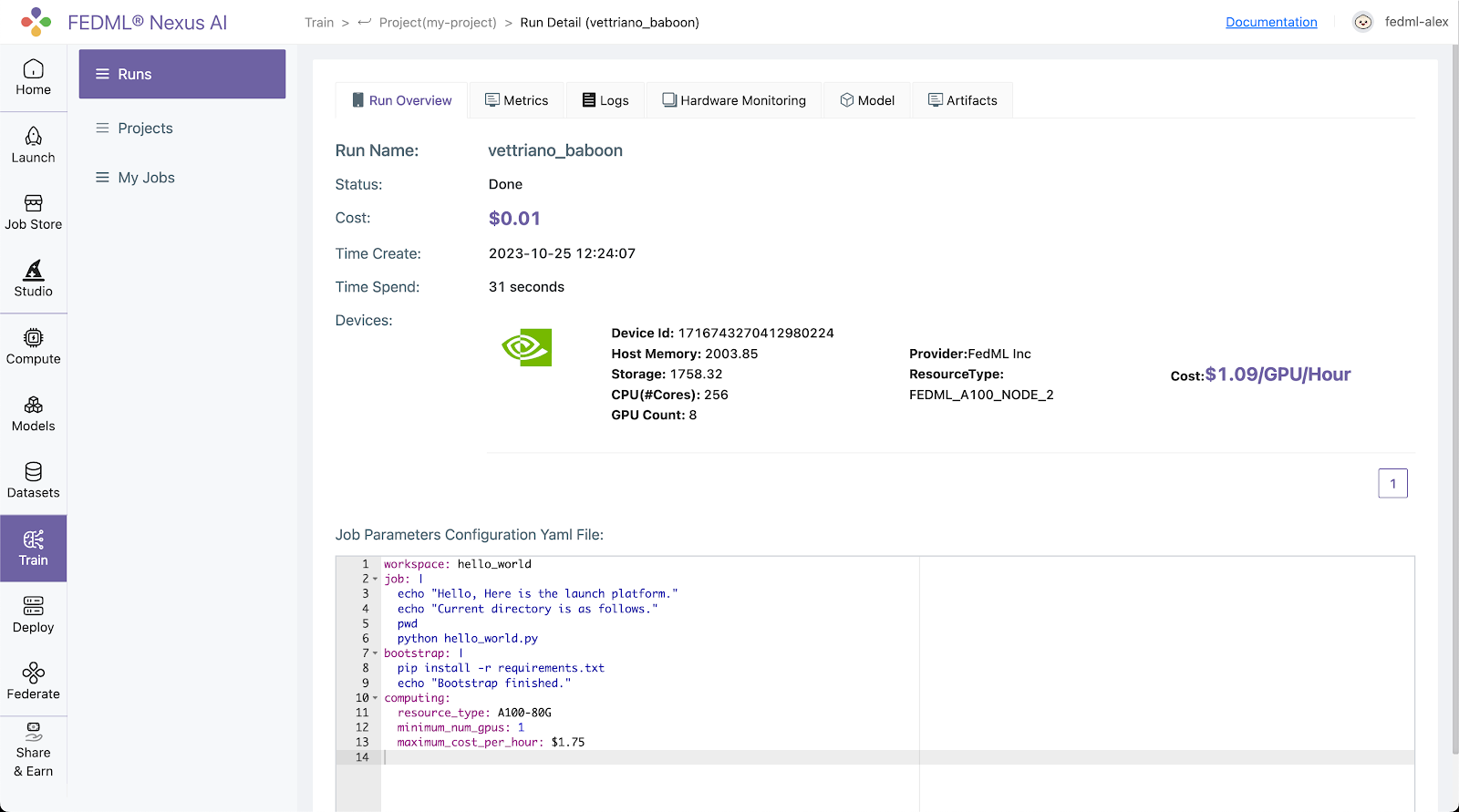
Training as a Cloud Service
FEDML Launch further enables “Training as a Cloud Service” at FEDML Nexus AI platform, providing a variety of GPU types (A100, H100, A6000, RTX4090, etc.) for developers to train your model at any time. Developers only pay per usage. It includes the following features:
- Cost-effective training: Developers do not need to rent or purchase GPUs, developers can initiate serverless training tasks at any time, and developers only need to pay according to the usage time;
- Flexible Resource Management: Developers can also create a cluster to use fixed machines and support the cluster autostop function (such as automatic shutdown after 30 minutes) to help you save the cost loss caused by forgetting to shut down the idle resources.
- Simplified Code Setup: You do not need to modify your python training source code, you only need to specify the path of the code, environment installation script, and the main entrance through the YAML file
- Comprehensive Tracking: The training process includes rich experimental tracking functions, including Run Overview, Metrics, Logs, Hardware Monitoring, Model, Artifacts, and other tracking capabilities. You can use the API provided by FEDML Python Library for experimental tracking, such as fedml.log
- GPU Availability: There are many GPU types to choose from. You can go to Secure Cloud or Community Cloud to view the type and set it in the YAML file to use it.
As an example of applying FEDML Launch for training service, LLM Fine-tune is the feature of FEDML Studio that is responsible for serverless model training. It is a no-code LLM training platform. Developers can directly specify open-source models for fine-tuning or model Pre-training.
Step 1. Select a model to build a new run
There are two choices for specifying the model to train:
- Select Default base model from Open Source LLMs
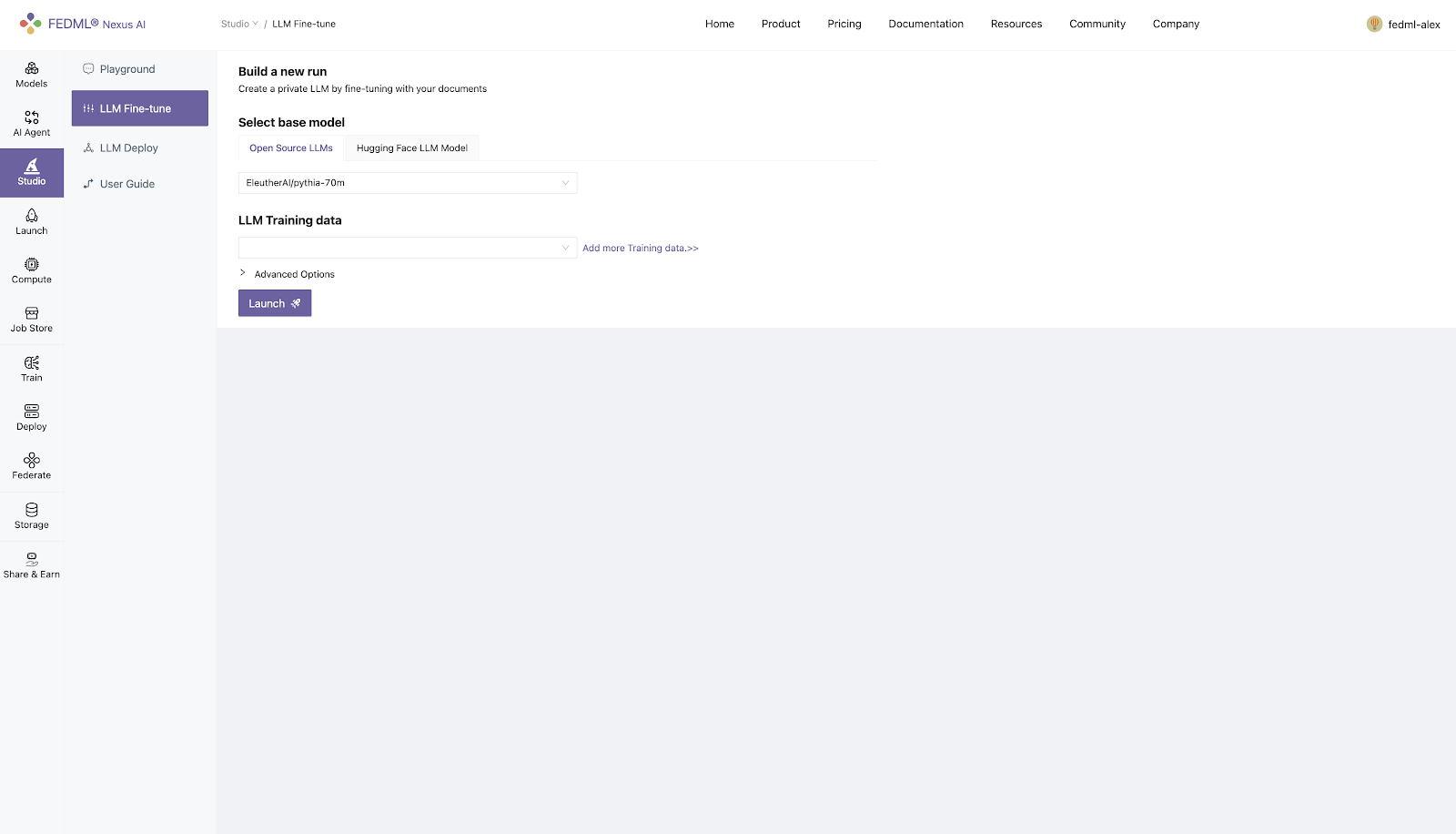
- Specifying HuggingFace LLM model path
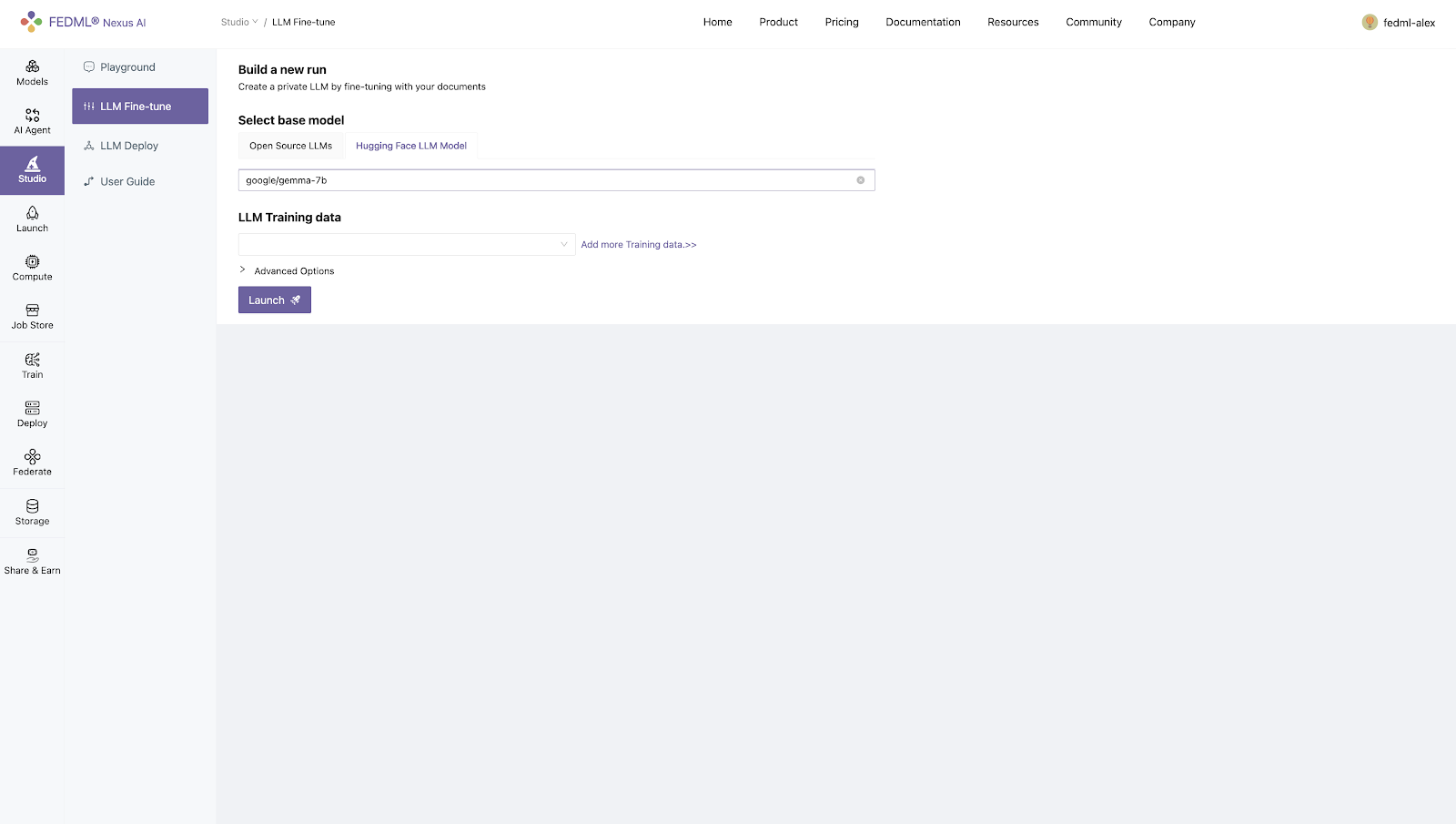
Step 2. Prepare training data
There are three ways to prepare the training data.
- Select the default data experience platform
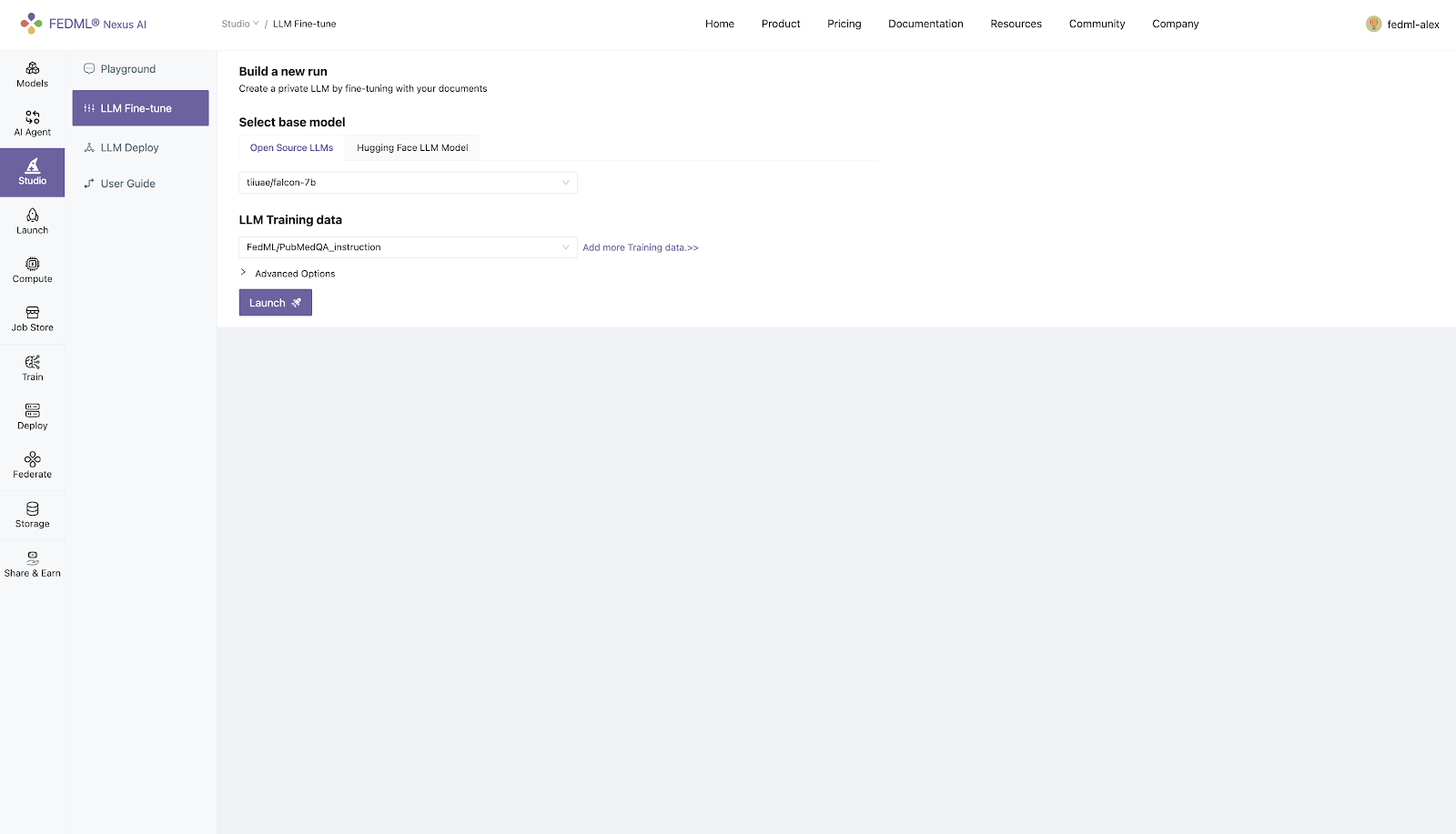
- Customized training data can be uploaded through the storage module
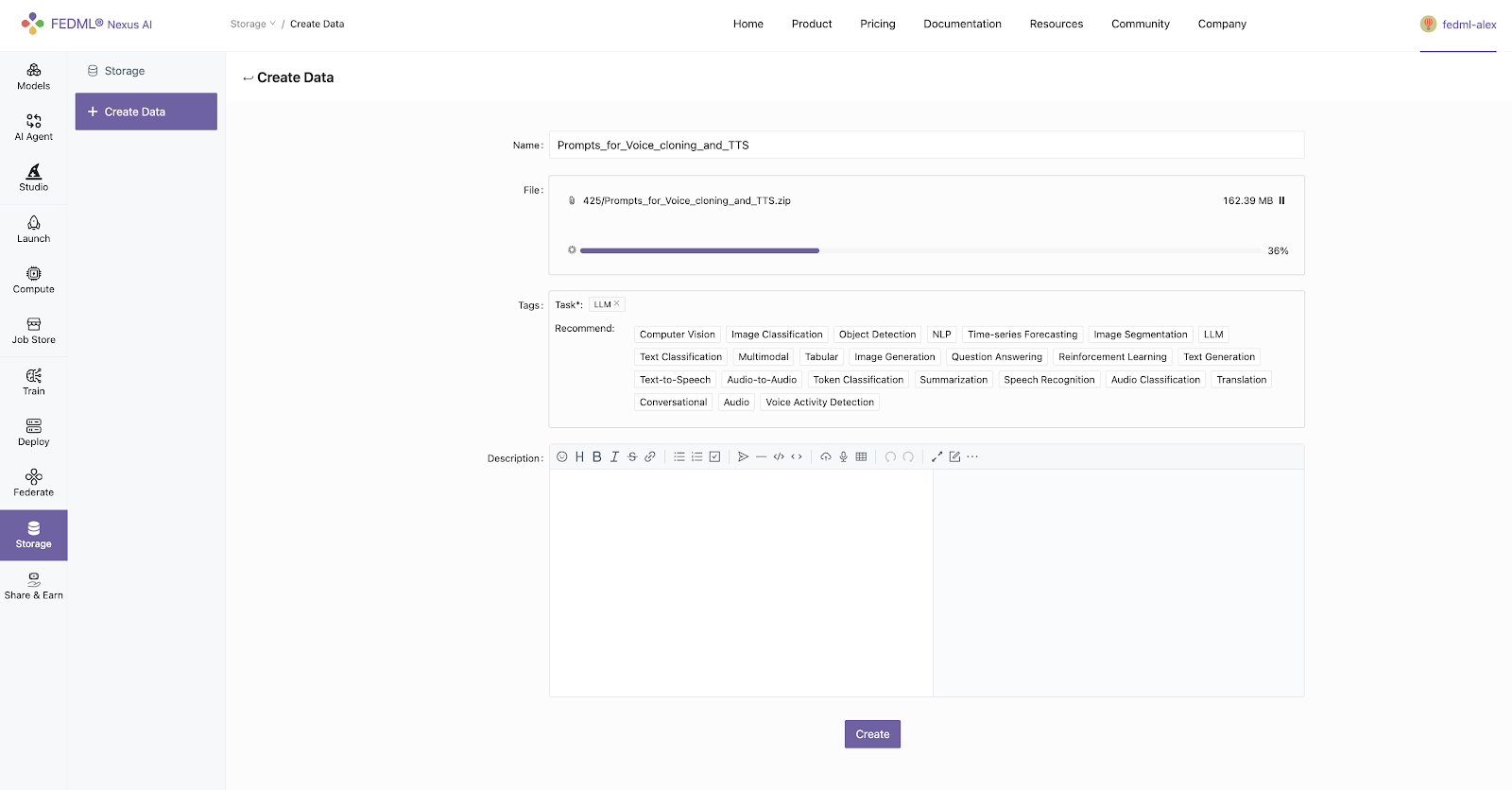
- Data upload API: fedml.api.storage
fedml storage upload '/path/Prompts_for_Voice_cloning_and_TTS'
Uploading Package to Remote Storage: 100%|██████████████████████████████████████████████████████████████████████████████████████████████████████████████████████████████████████████████████████████████████| 42.0M/42.0M [00:36<00:00, 1.15MB/s]
Data uploaded successfully. | url: https://03aa47c68e20656e11ca9e0765c6bc1f.r2.cloudflarestorage.com/fedml/3631/Prompts_for_Voice_cloning_and_TTS.zip?X-Amz-Algorithm=AWS4-HMAC-SHA256&X-Amz-Credential=52d6cf37c034a6f4ae68d577a6c0cd61%2F20240307%2Fus-east-1%2Fs3%2Faws4_request&X-Amz-Date=20240307T202738Z&X-Amz-Expires=604800&X-Amz-SignedHeaders=host&X-Amz-Signature=bccabd11df98004490672222390b2793327f733813ac2d4fac4d263d50516947
Step 3. Hyperparameter Setting (Optional)
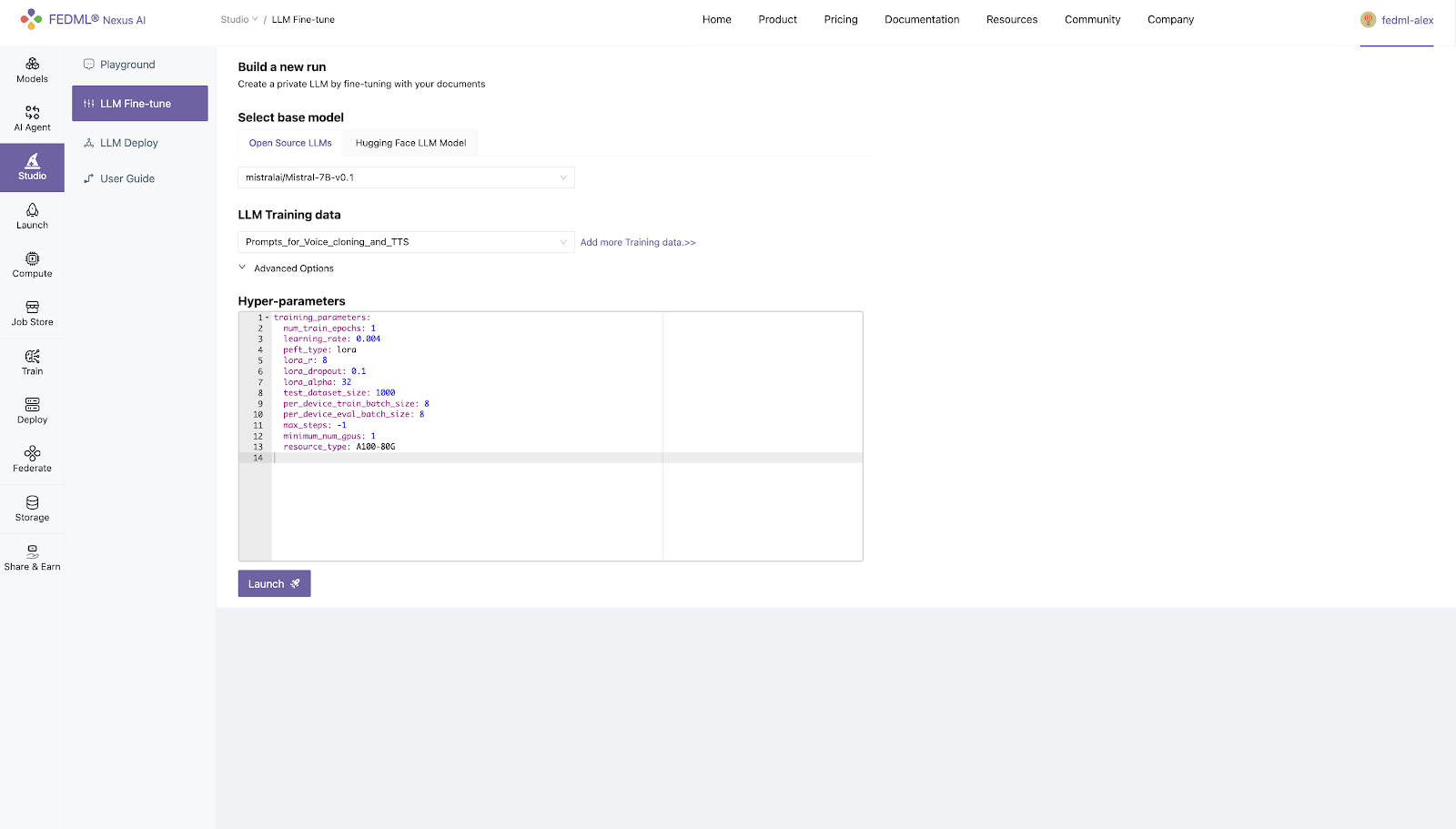
Step 4. Select GPU Resource Type (Optional)
The GPU resource type can be found through the Compute - Secure Cloud page
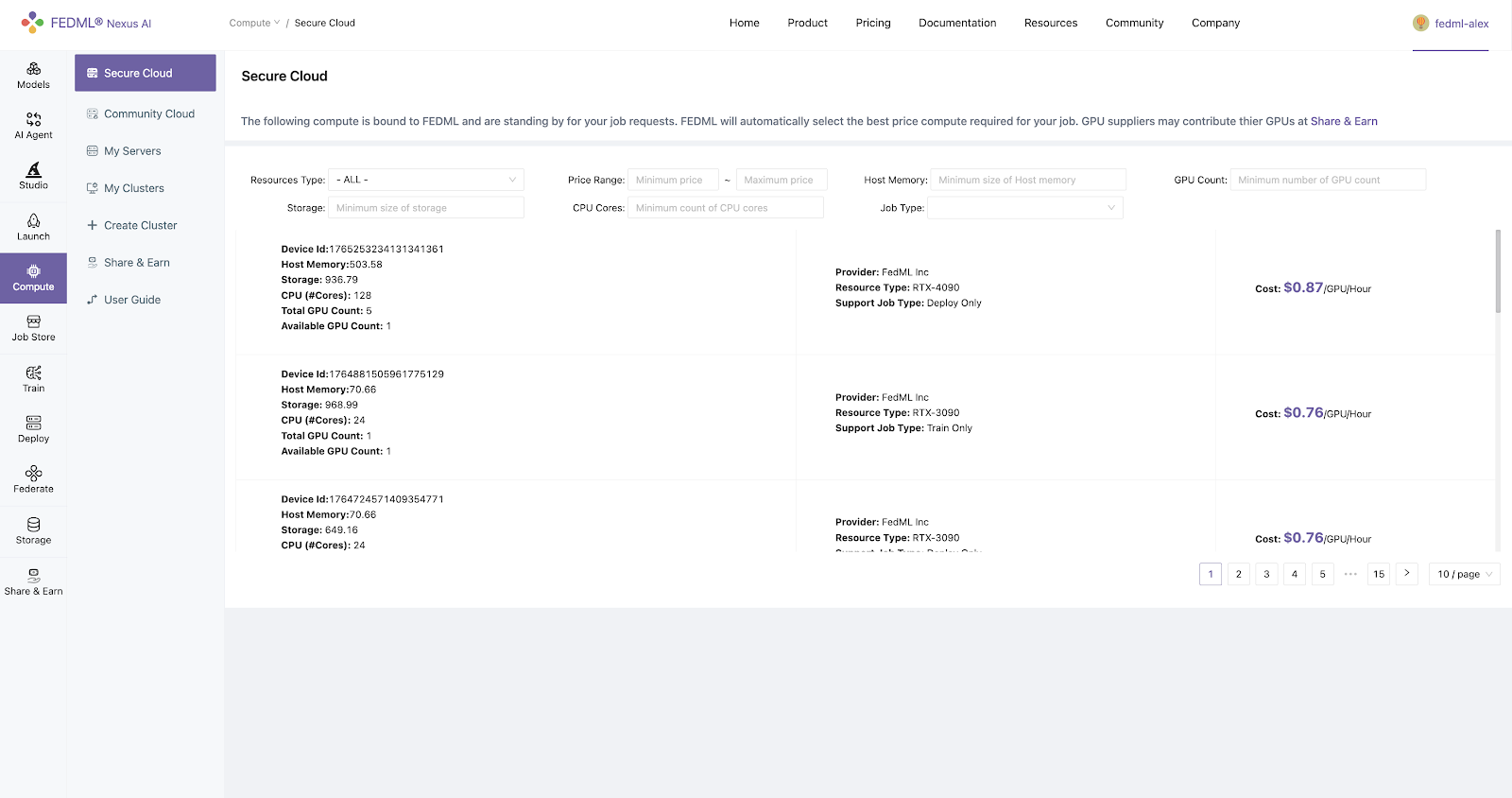
Step 5. Initiate Training and Track Experimental Results
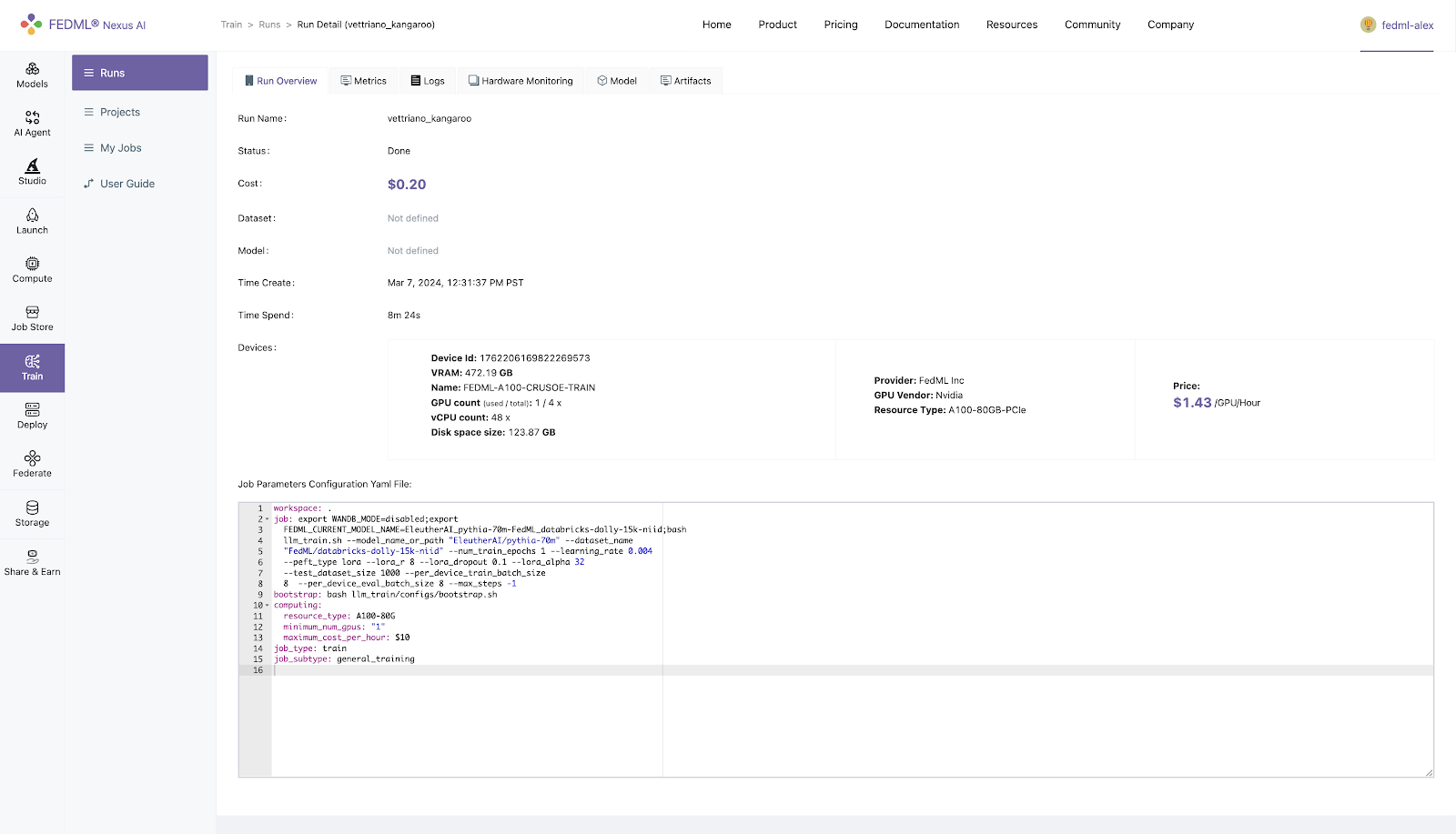
Train on Your Own GPU cluster
You can also build your own cluster and launch jobs there. The GPU nodes in the cluster can be GPU instances launched under your AWS/GCP/Azure account or your in-house GPU devices. The workflow is as follows.
Step 1. Bind the machines on the Platform
Log into the platform, head to the Compute / My Servers Page and copy the fedml login command:
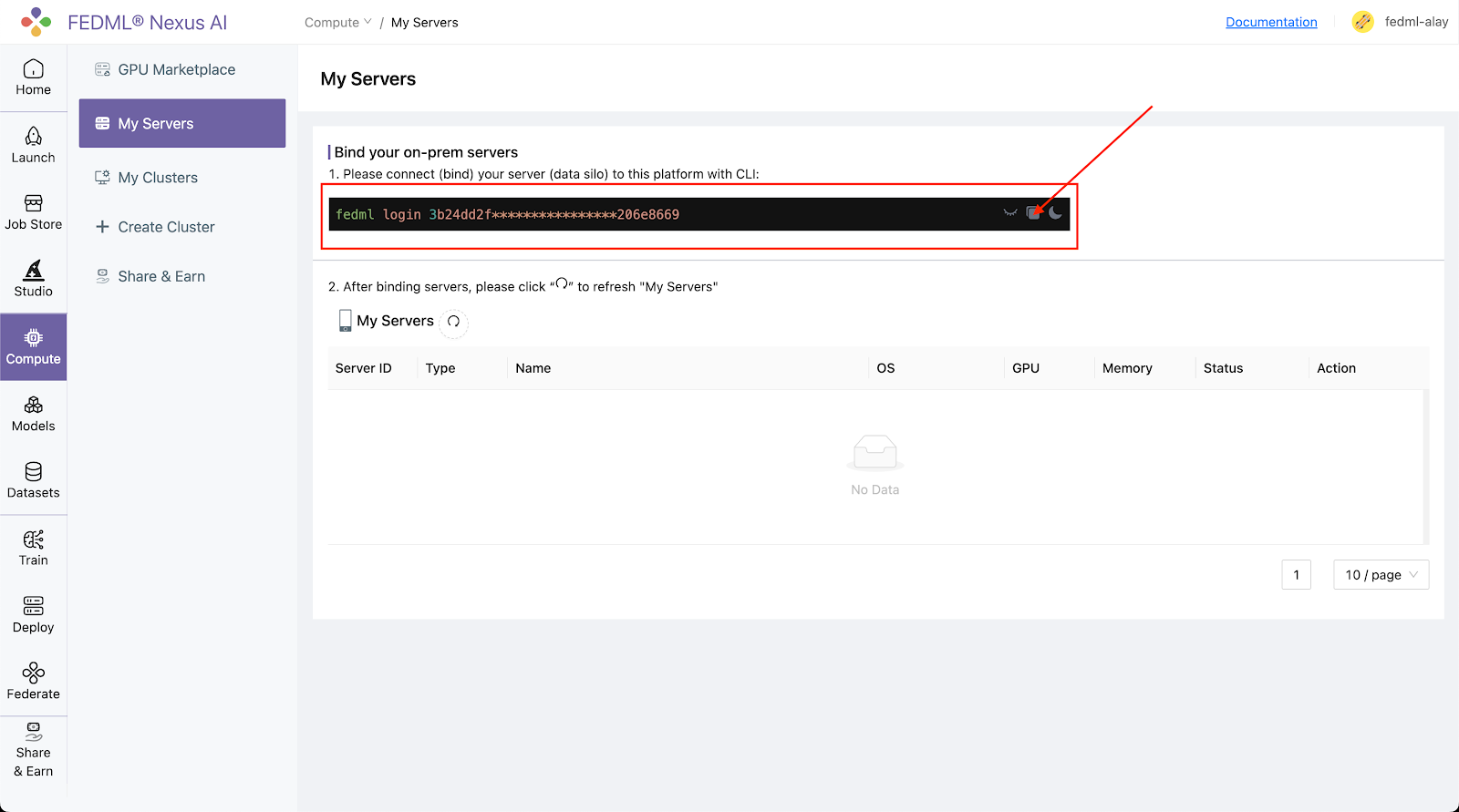
Step 2. SSH into your on-prem devices and do the following individually for each device:
Install the fedml library if not installed already:
pip install fedml
Run the login command copied from the platform:
fedml login 3b24dd2f****206e8669
It should show something similar as below:
(fedml) alay@a6000:~$ fedml login 3b24dd2f9b3e478084c517bc206e8669 -v dev
Welcome to FedML.ai!
Start to login the current device to the MLOps (https://fedml.ai)...
(fedml) alay@a6000:~$ Found existing installation: fedml 0.8.7
Uninstalling fedml-0.8.7:
Successfully uninstalled fedml-0.8.7
Looking in indexes: https://test.pypi.org/simple/, https://pypi.org/simple
Collecting fedml==0.8.8a156
Obtaining dependency information for fedml==0.8.8a156 from https://test-files.pythonhosted.org/packages/e8/44/06b4773fe095760c8dd4933c2f75ee7ea9594938038fb8293afa22028906/fedml-0.8.8a156-py2.py3-none-any.whl.metadata
Downloading https://test-files.pythonhosted.org/packages/e8/44/06b4773fe095760c8dd4933c2f75ee7ea9594938038fb8293afa22028906/fedml-0.8.8a156-py2.py3-none-any.whl.metadata (4.8 kB)
Requirement already satisfied: numpy>=1.21 in ./.pyenv/versions/fedml/lib/python3.10/site-packages (from fedml==0.8.8a156
.
.
.
.
Congratulations, your device is connected to the FedML MLOps platform successfully!
Your FedML Edge ID is 201610, unique device ID is 0xffdc89fad658@Linux.Edge.Device
Head back to the Compute / My Servers page on platform and verify that the devices are bounded to the FEDML Nexus AI Platform:
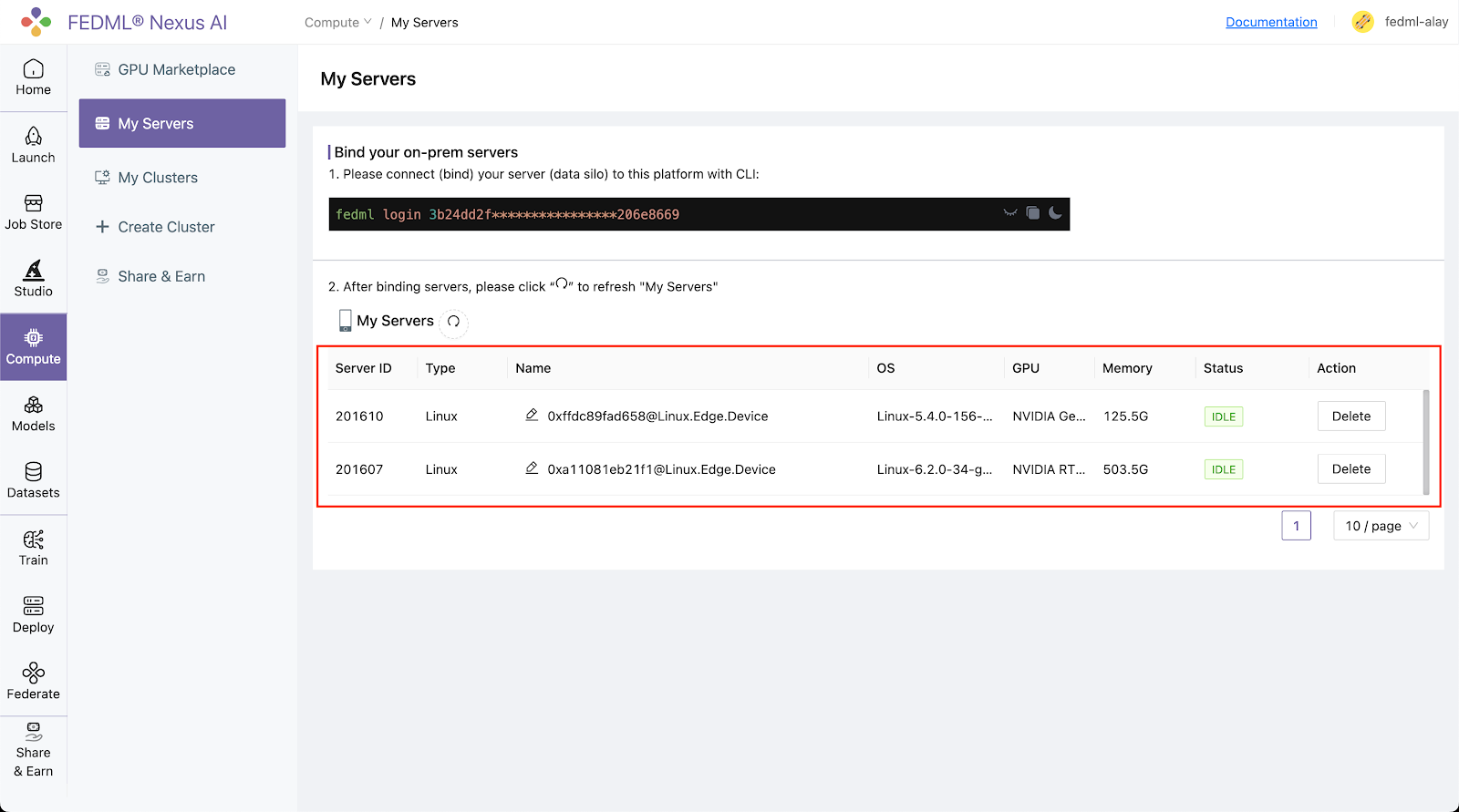
Step 3. Create a cluster of your servers bounded to the FEDML Nexus AI Platform:
Navigate to the Compute / Create Clusters page and create a cluster of your servers:
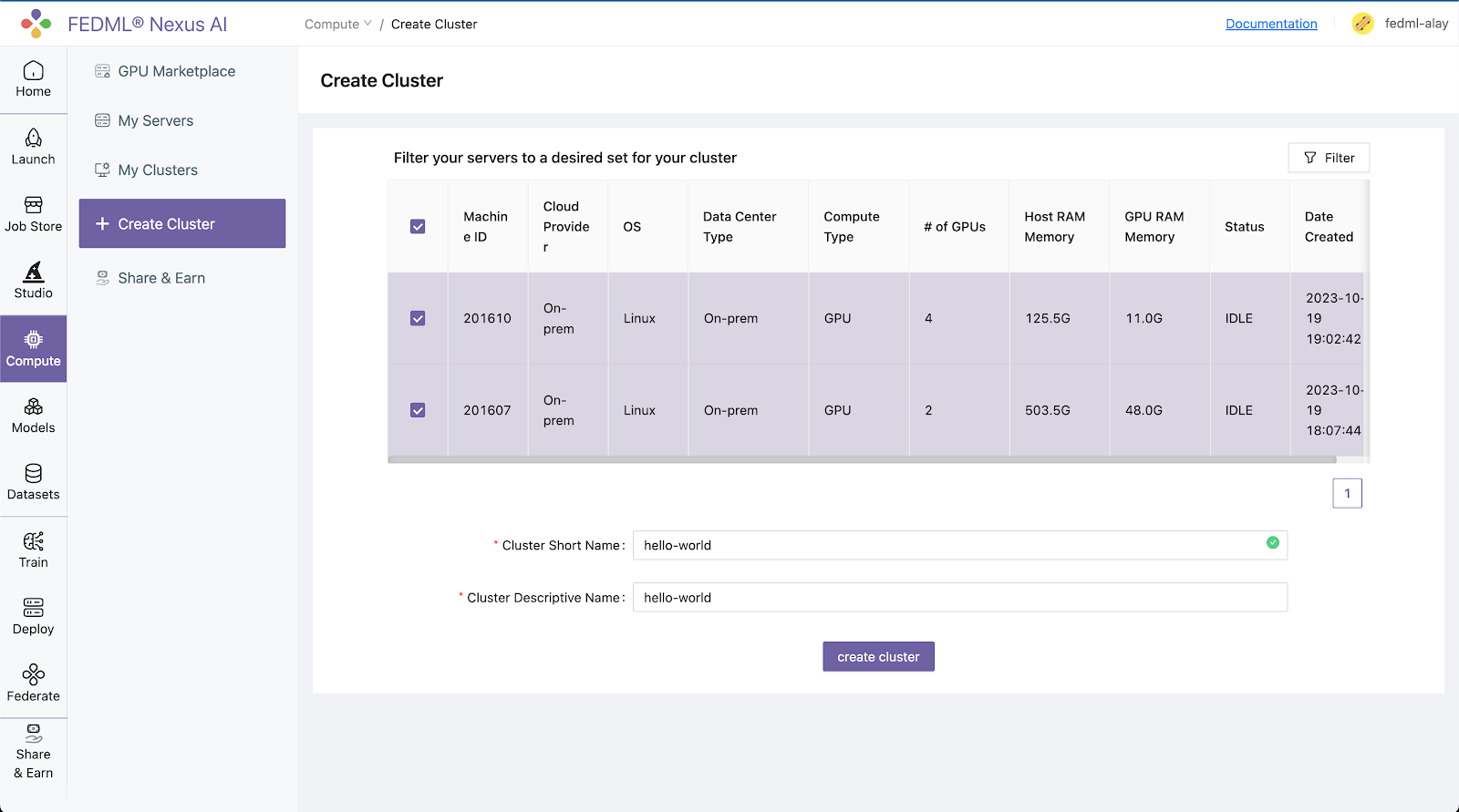
All your created clusters will be listed on the Compute / My Clusters page:
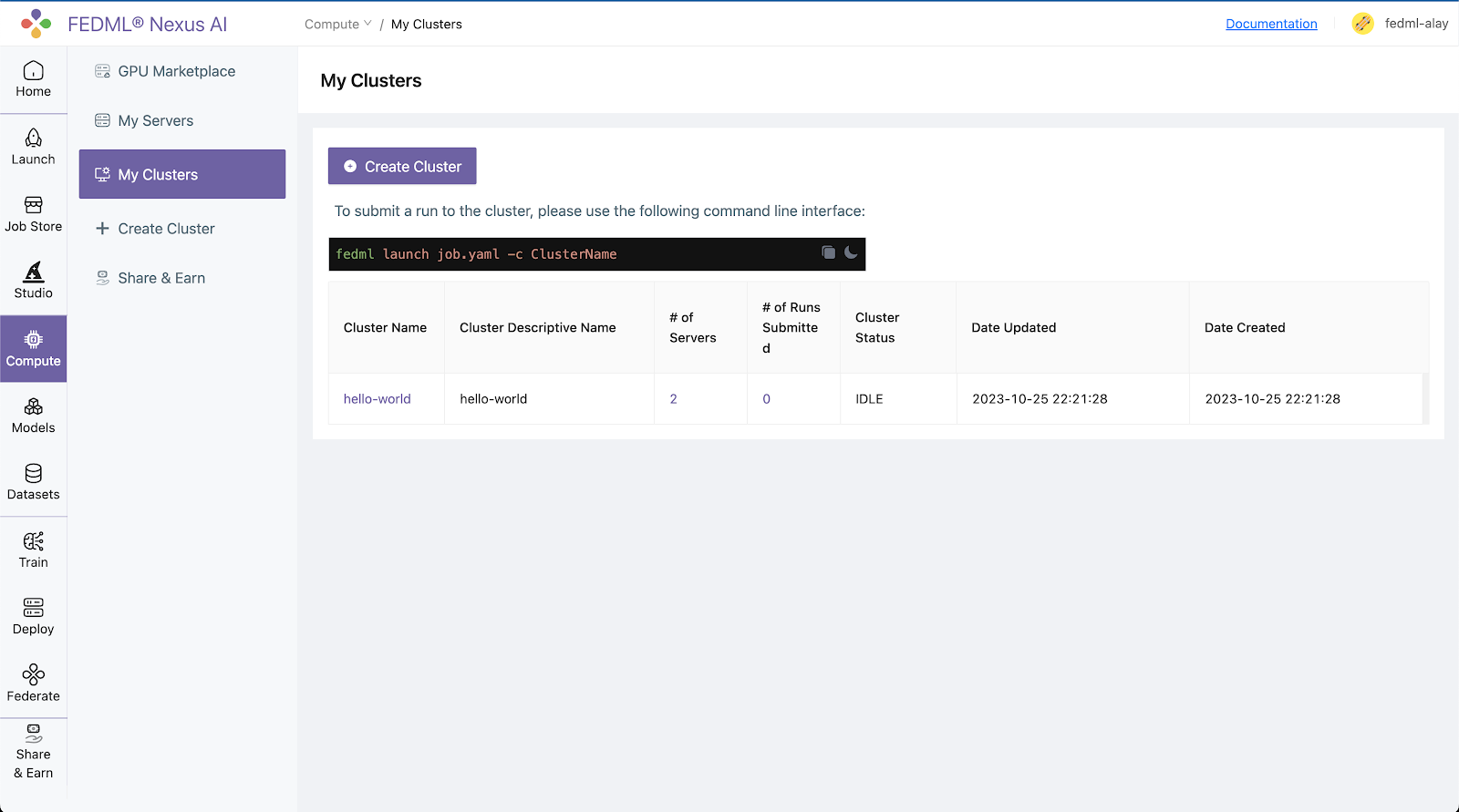
Step 4. Launch the job on your cluster:
The way to create the job YAML file is the same as “Training as a Cloud Service”. All that is left to do to launch a job to the on-premise cluster is to run following one-line command:
fedml launch job.yaml -c <cluster_name>
For our example, the command and respective output would be as follows:
fedml launch job.yaml -c hello-world
Experiment Tracking for FEDML Launch
Running remote tasks often requires a transparent monitoring environment to facilitate troubleshooting and real-time analysis of machine learning experiments. This section guides through the monitoring capabilities of a job launched using the “fedml launch” command.
Run Overview
Log into to the FEDML Nexus AI Platform (https://fedml.ai) and go to Train > Runs. And select the run you just launched and click on it to view the details of the run.
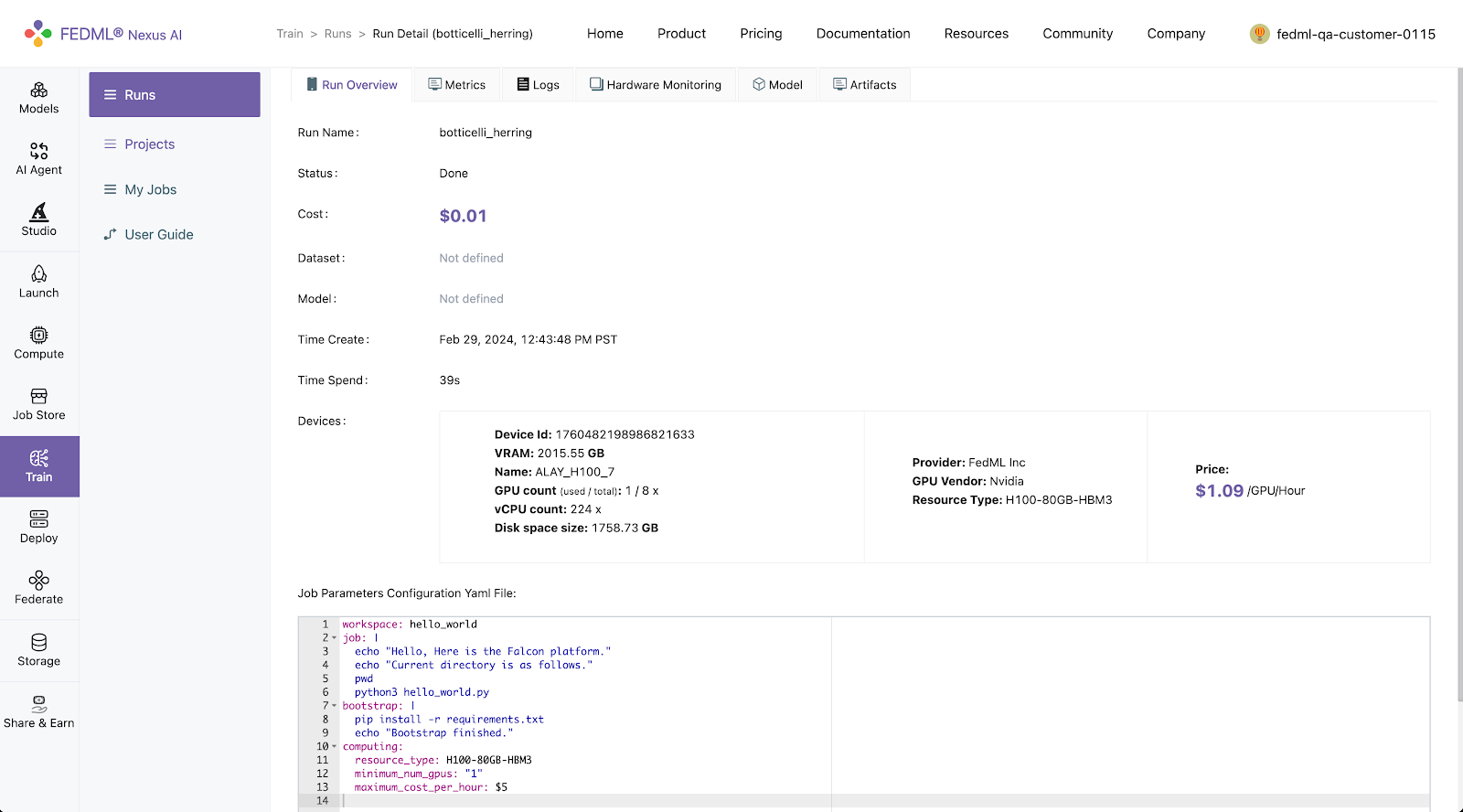
Metrics
FedML offers a convenient set of APIs for logging metrics. The execution code can utilize these APIs to log metrics during its operation.
fedml.log()
log dictionary of metric data to the FEDML Nexus AI Platform.
Usage
fedml.log(
metrics: dict,
step: int = None,
customized_step_key: str = None,
commit: bool = True) -> None
Arguments
- metrics (dict): A dictionary object for metrics, e.g., {"accuracy": 0.3, "loss": 2.0}.
- step (int=None): Set the index for current metric. If this value is None, then step will be the current global step counter.
- customized_step_key (str=None): Specify the customized step key, which must be one of the keys in the metrics dictionary.
- commit (bool=True): If commit is False, the metrics dictionary will be saved to memory and won't be committed until commit is True.
Example:
fedml.log({"ACC": 0.1})
fedml.log({"acc": 0.11})
fedml.log({"acc": 0.2})
fedml.log({"acc": 0.3})
fedml.log({"acc": 0.31}, step=1)
fedml.log({"acc": 0.32, "x_index": 2}, step=2, customized_step_key="x_index")
fedml.log({"loss": 0.33}, customized_step_key="x_index", commit=False)
fedml.log({"acc": 0.34}, step=4, customized_step_key="x_index", commit=True)
Metrics logged using fedml.log() can be viewed under Runs > Run Detail > Metrics on FEDML Nexus AI Platform.
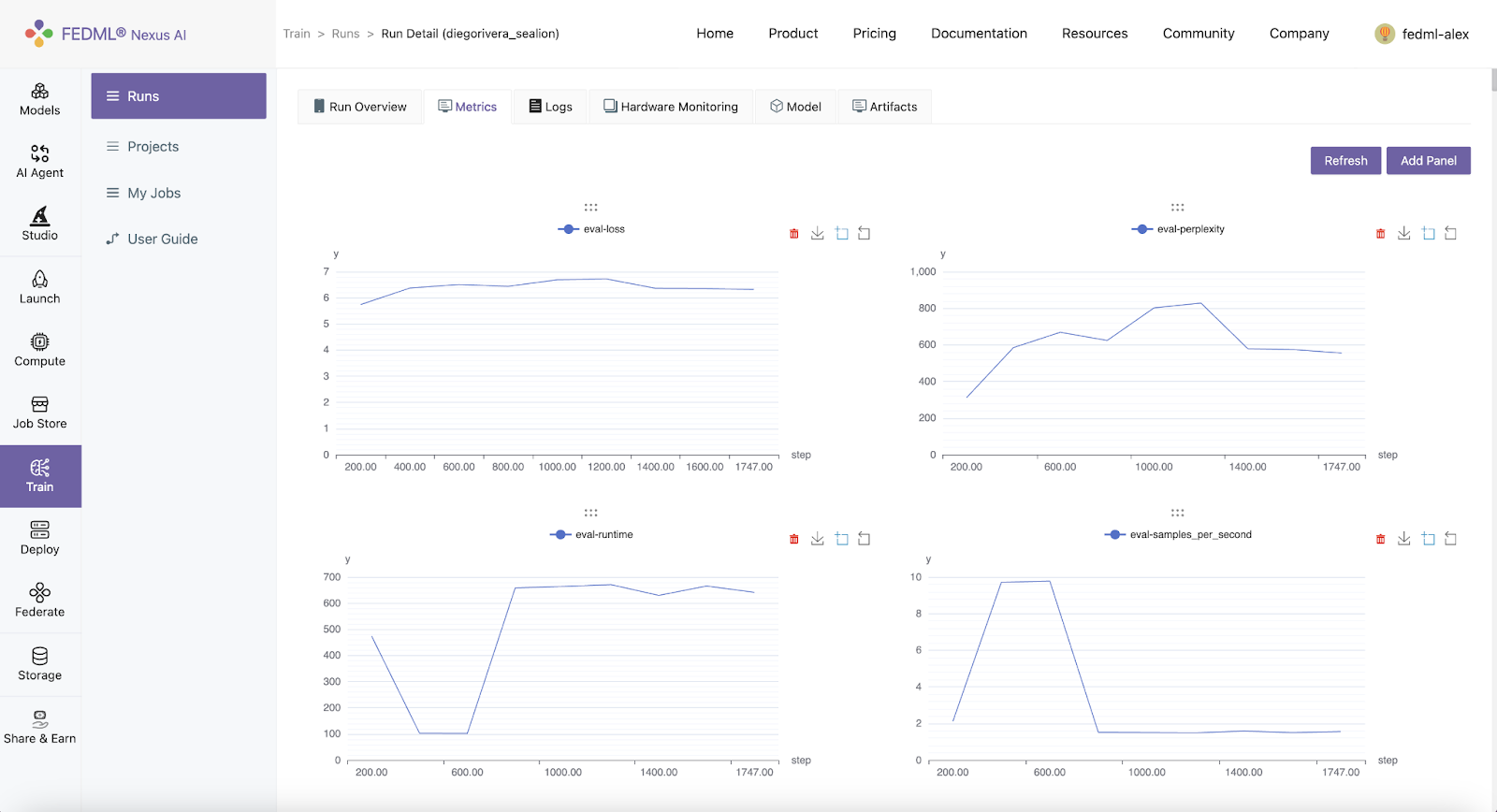
Logs
You can query the realtime status of your run on your local terminal with the following command.
fedml run logs -rid <run_id>
Additionally, logs of the run also appear in realtime on the FEDML Nexus AI Platform under the Runs > Run Detail > Logs
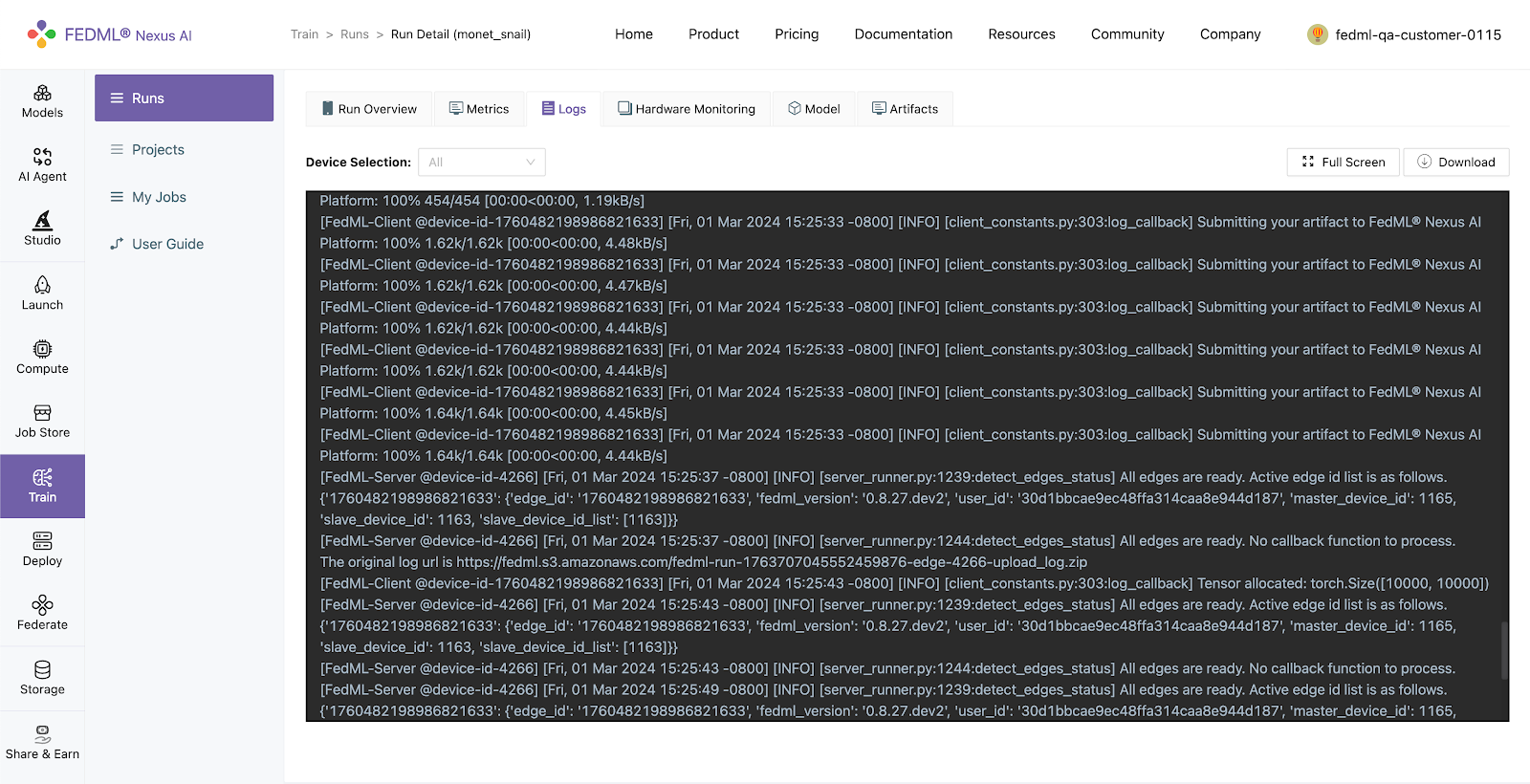
Hardware Monitoring
The FEDML library automatically captures hardware metrics for each run, eliminating the need for user code or configuration. These metrics are categorized into two main groups:
- Machine Metrics: This encompasses various metrics concerning the machine's overall performance and usage, encompassing CPU usage, memory consumption, disk I/O, and network activity.
- GPU Metrics: In environments equipped with GPUs, FEDML seamlessly records metrics related to GPU utilization, memory usage, temperature, and power consumption. This data aids in fine-tuning machine learning tasks for optimized GPU-accelerated performance.
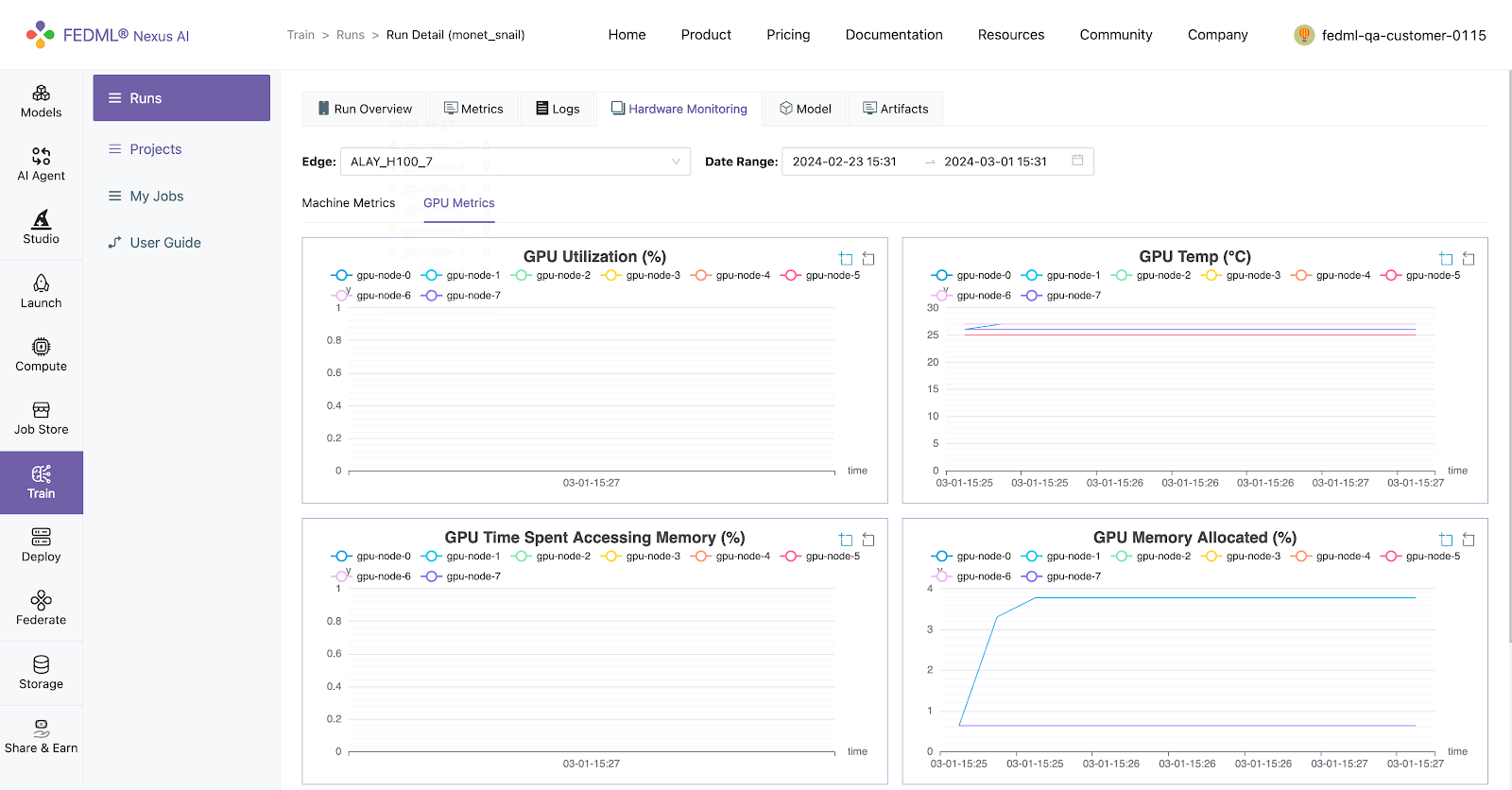
Model
FEDML additionally provides an API for logging models, allowing users to upload model artifacts.
fedml.log_model()
Log model to the FEDML Nexus AI Platform (fedml.ai).
fedml.log_model(
model_name,
model_file_path,
version=None) -> None
Arguments
- model_name (str): model name.
- model_file_path (str): The file path of model name.
- version (str=None): The version of FEDML Nexus AI Platform, options: dev, test, release. Default is release (fedml.ai).
Examples
fedml.log_model("cv-model", "./cv-model.bin")
Models logged using fedml.log_model() can be viewed under Runs > Run Detail > Model on FEDML Nexus AI Platform
Artifacts:
Artifacts, as managed by FEDML, encapsulate information about items or data generated during task execution, such as files, logs, or models. This feature streamlines the process of uploading any form of data to the FEDML Nexus AI Platform, facilitating efficient management and sharing of job outputs. FEDML facilitates the uploading of artifacts to the FEDML Nexus AI Platform through the following artifact api:
fedml.log_artifact()
log artifacts to the FEDML Nexus AI Platform (fedml.ai), such as file, log, model, etc.
fedml.log_artifact(
artifact: Artifact,
version=None,
run_id=None,
edge_id=None) -> None
Arguments
- artifact (Artifact): An artifact object represents the item to be logged, which could be a file, log, model, or similar.
- version (str=None): The version of FEDML Nexus AI Platform, options: dev, test, release. Default is release (fedml.ai).
- run_id (str=None): Run id for the artifact object. Default is None, which will be filled automatically.
- edge_id (str=None): Edge id for current device. Default is None, which will be filled automatically.
Artifacts logged using fedml.log_artifact() can be viewed under Runs > Run Detail > Artifactson FEDML Nexus AI Platform.
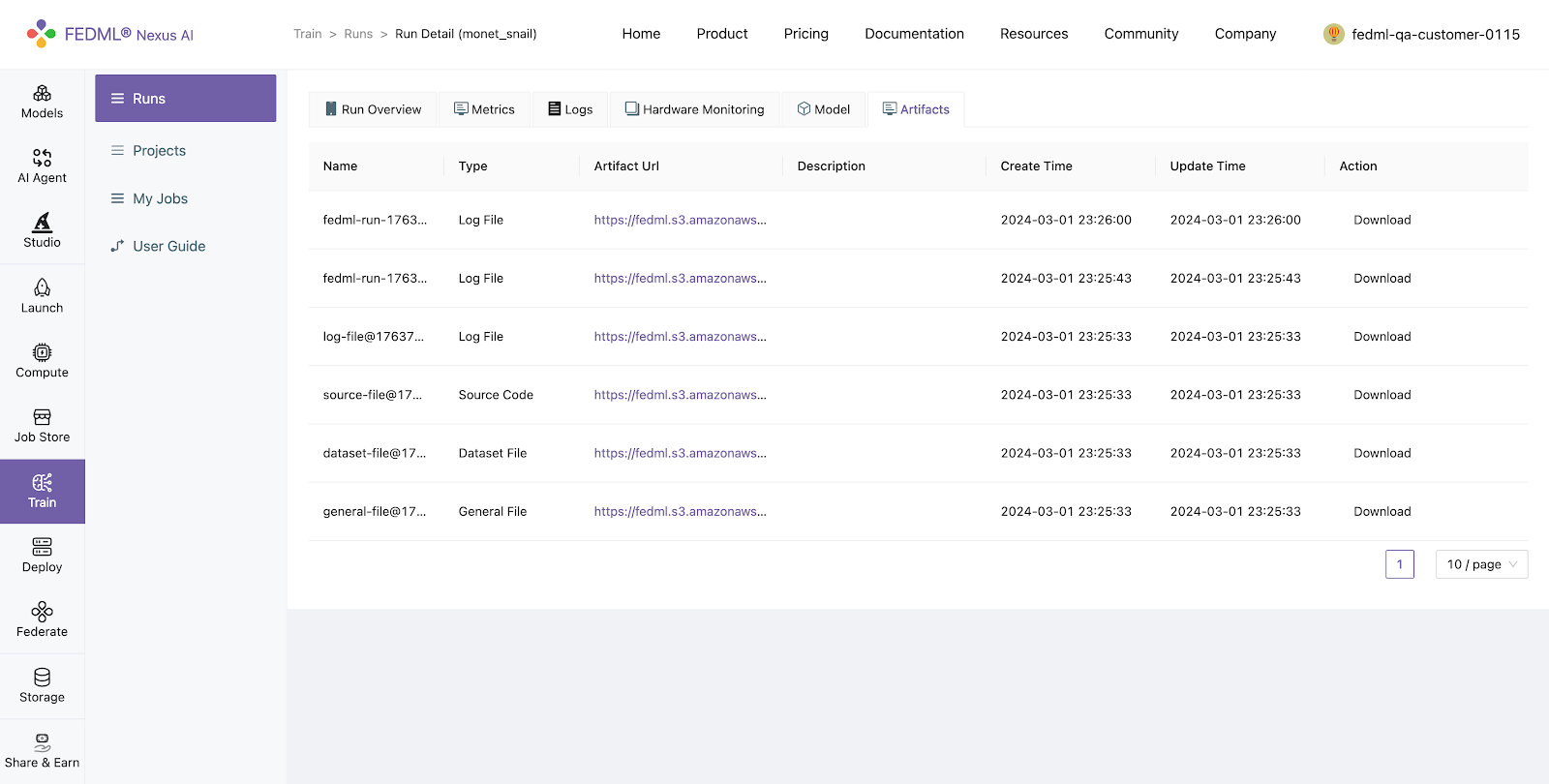
Advanced Features
FEDML Launch has numerous advanced features, which we plan to explore in depth in a forthcoming article. For now, we highlight a selection of key functionalities.
Batch Jobs
Batch Job is an advanced feature of FEDML Launch. It is designed for managing high-concurrency, multi-user training job queues. It distributes these jobs across decentralized GPU clusters, optimizing scalability, throughput, and achieving rapid task digestion and high GPU utilization, thereby improving generative AI user experience and GPU cost.
The applicable scenarios include:
- A large number of internet users initiate fine-tuning or inference tasks concurrently in a short period.
- Team members manage submitted concurrent tasks within their self-hosted GPU cluster.
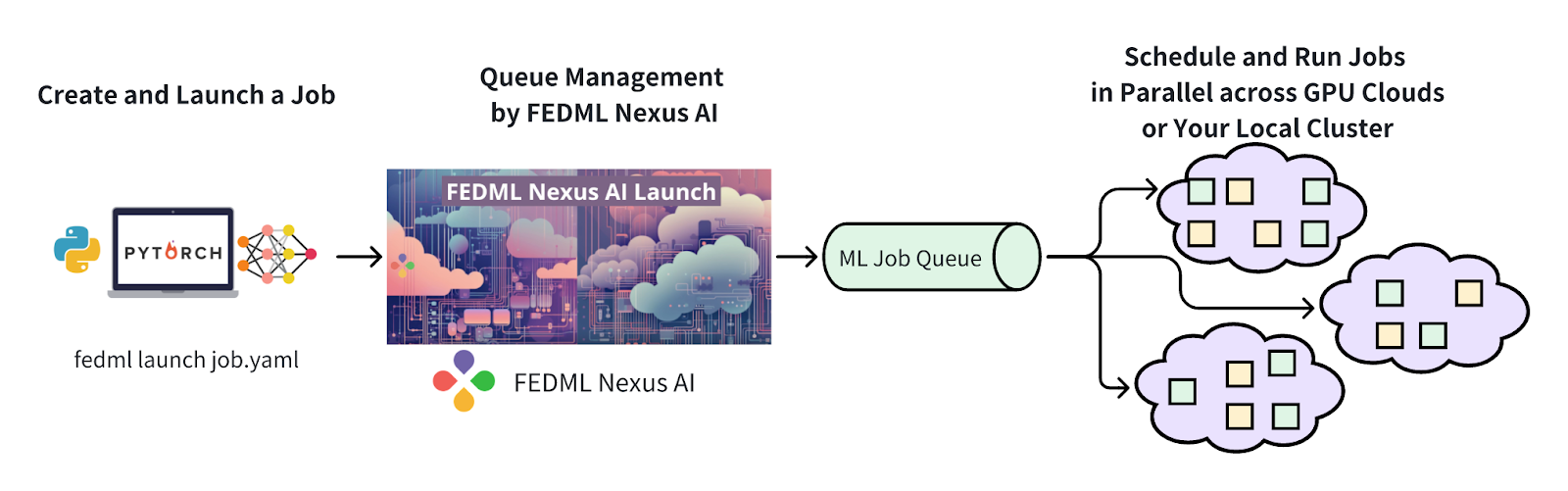
Developers only need to launch a large number of jobs through CLI or API such as FEDML launch job.yaml, then the FEDML Launch will go for complex scheduling and experiment management.
Workflow for compound training and serving jobs
Besides managing batch jobs, there's often a need to integrate various training and serving tasks into comprehensive ML pipelines. Typical scenarios include:
- Establishing a pipeline spanning from data collection to training, fine-tuning, serving, and model improvement.
- Orchestrating tasks where one job performs initial work and then passes data to another job, which may invoke an inference endpoint, resembling a workflow.
This is where the FEDML Workflow API proves valuable. FEDML Launch Workflow API is a user-friendly interface for defining jobs and their dependencies, leveraging the underlying FEDML core fleet of APIs.
About FEDML, Inc.
FEDML is your generative AI platform at scale to enable developers and enterprises to build and commercialize their own generative AI applications easily, scalably, and economically. Its flagship product, FEDML Nexus AI, provides unique features in enterprise AI platforms, model deployment, model serving, AI agent APIs, launching training/Inference jobs on serverless/decentralized GPU cloud, experimental tracking for distributed training, federated learning, security, and privacy.
FEDML, Inc. was founded in February 2022. With over 5000 platform users from 500+ universities and 100+ enterprises, FEDML is enabling organizations of all sizes to build, deploy, and commercialize their own LLMs and Al agents. The company's enterprise customers span a wide range of industries, including generative Al/LLM applications, mobile ads/recommendations, AloT (logistics/retail), healthcare, automotive, and web3.The company has raised $13.2M seed round. As a fun fact, FEDML is currently located at the Silicon Valley "Lucky building" (165 University Avenue, Palo Alto, CA), where Google, PayPal, Logitech, and many other successful companies started.